Enhancing Water Dynamics Modeling: The Intersection of Physics-Based Models and AI
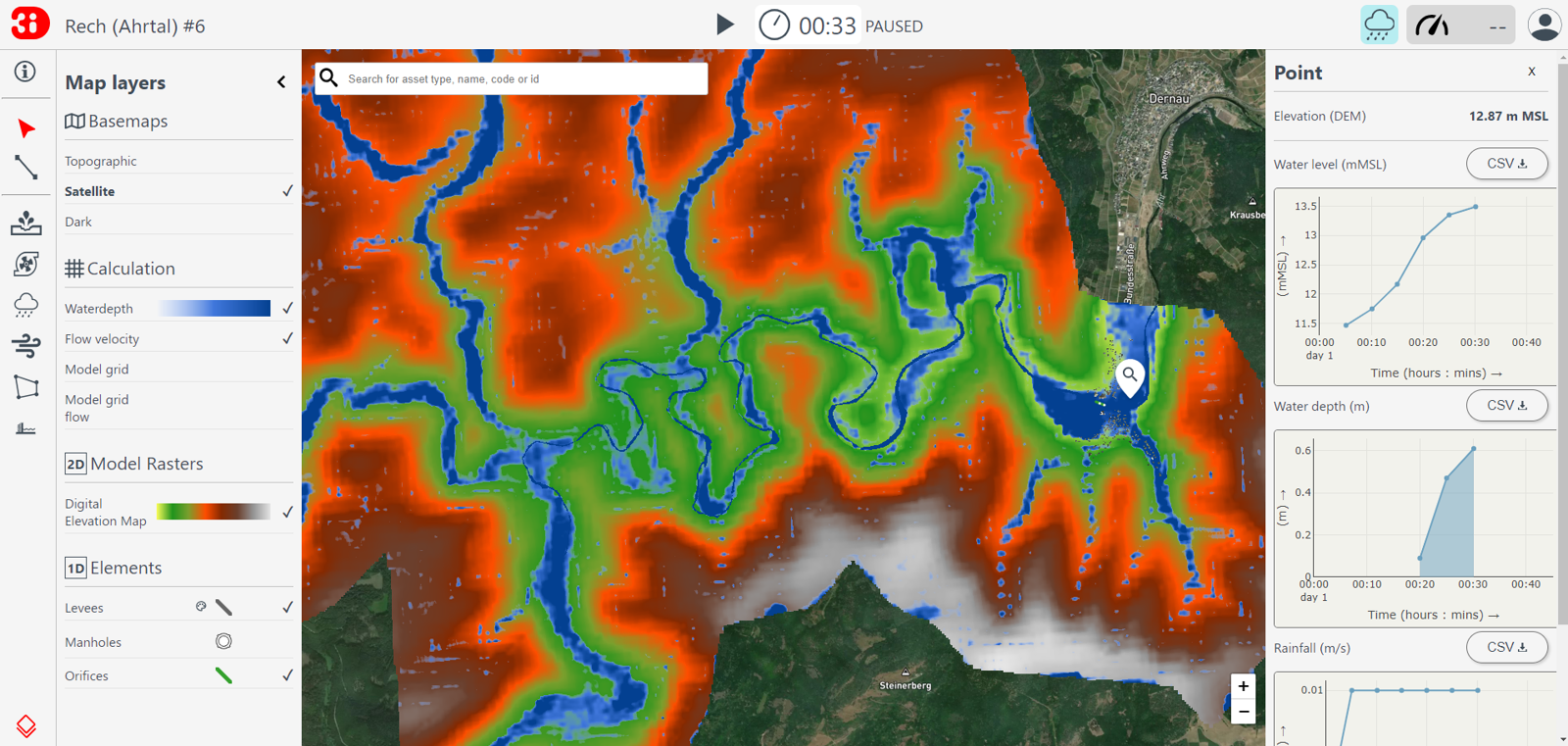
9 January 2024
J. van Schrojenstein Lantman MSc, Nelen&Schuurmans Technology BV.
Drs J.Grispen, Nelen&Schuurmans Technology BV.
Introduction
To effectively address the multifaceted challenges posed by climate change, it is crucial to comprehend its various dimensions. In particular, the impact of climate change is closely linked to water, accounting for 90% of its consequences. The extremes of excessive water, resulting in flooding, and insufficient water, causing drought, have a substantial impact on both food security and water availability. Therefore, a thorough understanding of water dynamics becomes imperative for effective adaptation and mitigation strategies.
Potential advantages of AI models
Water experts have long benefited from the support of physics-based numerical models in their research endeavours. However, in the current era, there is a growing need to explore the applicability and added value of AI over traditional physics-based models. Examining the potential advantages of using AI, reveals several noteworthy points:
- Complexity and non-linearity:
AI excels in handling complex and non-linear relationships, providing a valuable tool for understanding intricate water dynamics. Despite the well-known and well-described nature of water dynamics, the sheer scale of the problem introduces complexity, presenting an opportunity for AI.
- Data-Driven Approach:
AI models leverage diverse datasets, outperforming physics-based models that rely on explicit equations. Properly training an AI model on hydrodynamics needs diverse data types, including physical data, environmental data, historical data, and sensor data. The difficulty, however, relies on the lack of sufficient data for more than half the globe. Although satellite data can help address this gap, its limited resolution constrains the applicability of AI models trained with such information.
- Adaptability to Real-World Complexity:
In real-world scenarios, where systems can be highly complex, AI models adapt to the intricacies of diverse datasets. This adaptability is particularly relevant in urban areas prone to flooding, where AI can effectively navigate the complexities introduced by the infrastructure above (buildings, roads, bridges) and below (sewerage) the surface.
- Cost-Effective Training:
Training AI models prove to be less resource-intensive than developing and solving complex physics-based equations, especially for high-dimensional or chaotic systems. The time-consuming calibration and validation required for physics-based models further highlights the advantage of AI.
Hybrid modelling
While AI models offer potential advantages, they encounter pitfalls such as data quality and availability issues, model overfitting, scale and resolution challenges, and susceptibility to changing environmental conditions. To overcome these issues and ensure that water experts have reliable information, a widely recommended approach involves adopting a hybrid modelling strategy (1) that integrates both AI and physics-based models.
Strengthening Hybrid Modelling
There are several ways to strengthen hybrid modelling. Physics-based models, for example, can generate simulation data as a novel data type to effectively train the AI model, thereby addressing the issues related to data quality and availability. By increasing the size and diversity of the training data, one can better generalise and minimise the possibility of model overfitting.
Connect models of various types in such a way that the generated output of one is the input for the other. This provides the expert with more freedom to apply the right model for the right scale and resolution issues. Keeping in mind that this type of modelling needs platform technology that supports hybrid modelling and can be fed by real-time data to meet the challenge of changing environmental conditions.
The TEMA project
In conclusion, considering the evolving challenges in water dynamics modelling, the collaboration of physics-based models and AI is pivotal. The TEMA project serves as a unique environment for extensive research in the field, bringing together suppliers of physics-based modelling software and AI science institutes. This collaborative effort, supported by four pilot regions, aims not only for theoretical advancements but also practical applications in real-life situations. The open sharing of results contributes to a better understanding and improved multi-hazard risk management.
Footnote:
(1) A large number of reviews and editorials describe hybrid simulation as the main challenge for data and AI-based simulation within the geosciences (Irrgang et al, 2021; Nearing et al, 2021; Razavi et al, 2022; Reichstein et al, 2019; C. Shen et al, 2021).