Enhancing Disaster Preparedness through Synthetic Data Generation in AI and Machine Learning
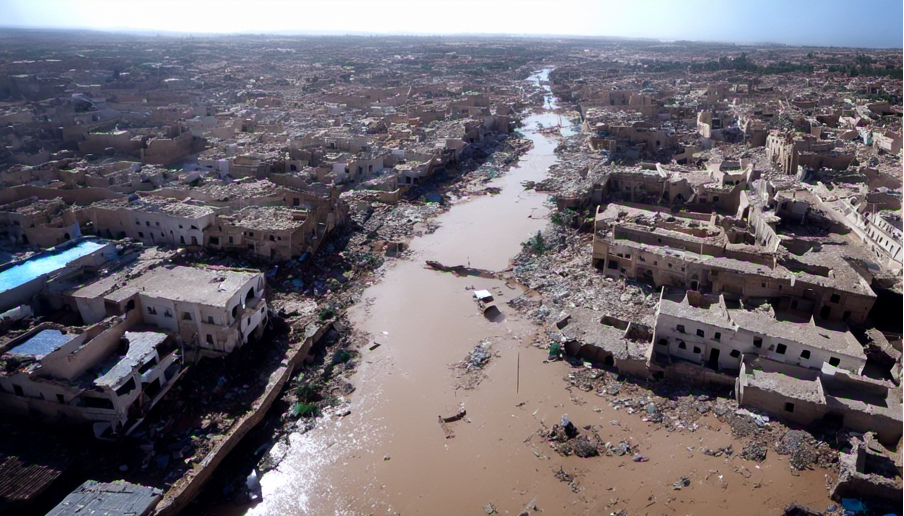
2 November 2023
Eleonor Diaz, Computer Vision Engineer at Atos Spain.
Data is often considered the lifeblood of machine learning algorithms. The availability of vast amounts of data has played a vital role in the rapid development of AI applications across various domains. However, a significant challenge that researchers and practitioners face in the AI landscape is the issue of data scarcity. Data scarcity refers to the limited availability or inadequacy of high-quality data for training AI models. This problem can arise from different circumstances:
- Limited Quantity: One of the most common aspects of data scarcity is the insufficient volume of data available for training AI models. Machine learning algorithms often require massive datasets to generalize well and make accurate predictions. In cases where relevant data is scarce, models may struggle to achieve acceptable performance.
- Imbalanced Data: Imbalanced datasets occur when certain classes or categories in the data are significantly underrepresented compared to others. This can lead to biased models that perform poorly on minority classes and important but rare events.
- Low Quality: Data quality is essential for training effective AI models. Noisy, inaccurate, or incomplete data can lead to erroneous predictions and suboptimal performance. In some cases, data scarcity results from a lack of high-quality, reliable data.
One approach to addressing data scarcity comes from Synthetic Data Generation. In certain scenarios, it is possible to generate synthetic data that closely resembles real-world data. Generative models, such as Generative Adversarial Networks (GANs), can create synthetic data points that expand the training dataset.
Synthetic data generation aids in improving the robustness of machine learning models used for natural disaster detection. By exposing these models to a wide range of synthetic scenarios, they become better equipped to handle unexpected or extreme situations during real disasters. Synthetic data can help in creating datasets for novel or rare disaster types that may not have sufficient historical data available, enabling early warning systems to be more comprehensive and effective.
Also, collecting real-world data for such scenarios can be a resource-intensive and time-consuming process, requiring extensive fieldwork, sensor deployments, and data acquisition infrastructure. In contrast, generating synthetic data is a relatively cost-effective and rapid solution. This cost-efficiency not only accelerates the development of predictive models and early warning systems but also frees up resources for other critical aspects of disaster preparedness and response.
In the past month, we witnessed one of the deadliest and most disastrous Mediterranean tropical cyclones in recent history, Storm Daniel, as it affected Bulgaria, Greece, Libya, and Turkey. In the city of Derna in Libya alone, there are at least 5,300 deaths and more than 10,000 people missing. It suffered heavy rains and floods that caused the destruction and collapse of around one-quarter of the city [1].
We were able to produce the following synthetic images that mimic the horrific situation currently occurring in Derna. The resulting images are quite photorealistic and share similar characteristics to real ones. These images could be implemented to improve the accuracy of a people detector model for a Search and Rescue application.
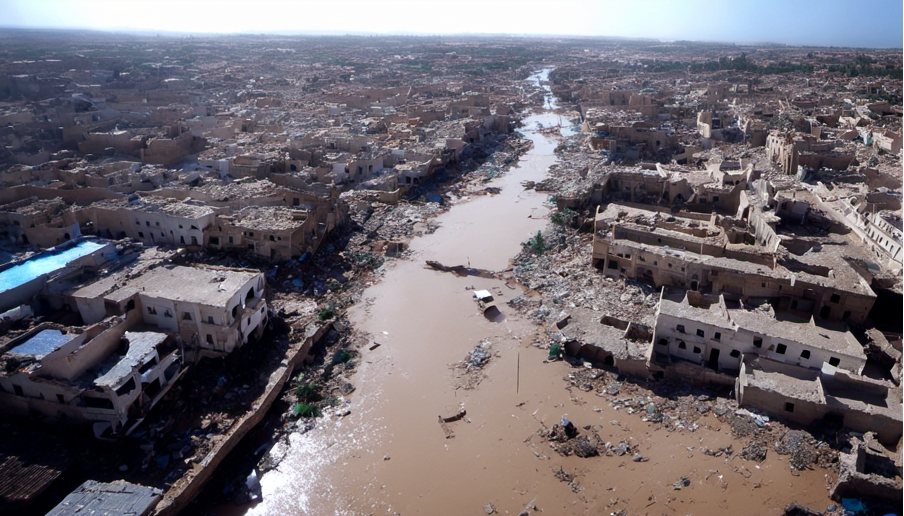
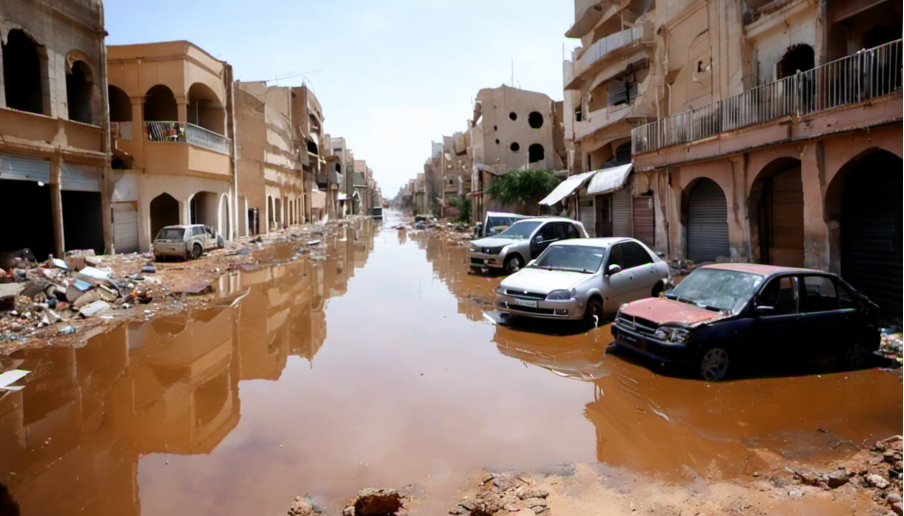
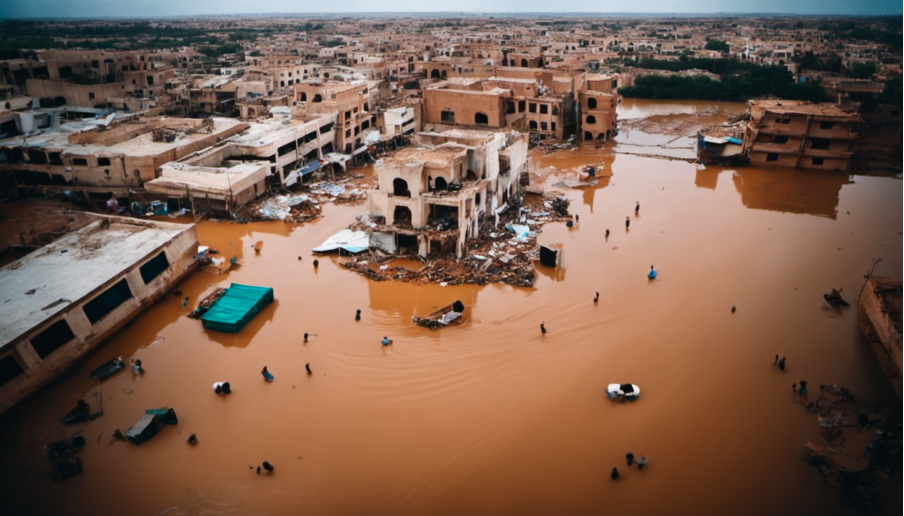
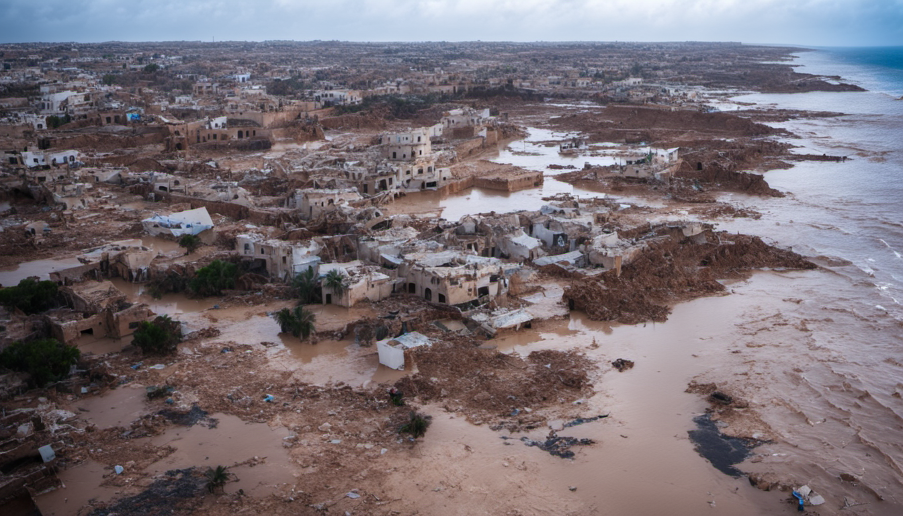
As we witness the devastating impact of such natural disasters, it underscores the urgency of improving our predictive models and early warning systems. We were able to produce synthetic images that mimic the horrific situation currently happening in Derna.
These synthetic images hold the potential to significantly improve the accuracy of people detection models used in Search and Rescue applications. During large-scale disasters like Storm Daniel, the ability to swiftly locate and assist individuals in distress is paramount. By incorporating synthetic data into the training process, we can ensure that these models are better prepared to handle the unique challenges posed by such events.
In conclusion, synthetic data generation stands as a powerful tool in addressing data scarcity in the field of AI and machine learning, particularly in contexts where real-world data is limited, expensive to acquire, or impractical to collect. As we continue to develop and refine AI applications for disaster management, synthetic data generation remains a valuable ally, helping us build more resilient, efficient, and effective solutions for a safer and more secure future.
Bibliography:
[1]Wintour, Patrick, and Patrick Wintour Diplomatic editor. “Libya: 10,000 Missing after Unprecedented Floods, Says Red Cross.” The Guardian, 12 Sept. 2023, www.theguardian.com/world/2023/sep/12/libya-floods-death-toll-dams-burst.
[2]Wintour, Patrick. “Libya: Thousands Missing after Dam Collapse Causes Massive Flooding.” Www.youtube.com, 12 Sept. 2023, youtu.be/SloqB7dPE0s. Accessed 28 Sept. 2023.
[3]Wintour, Patrick . “Libya: “Entire Neighbourhoods Disappeared” after Deadly Flooding, Say Officials.” Www.youtube.com, 12 Sept. 2023, youtu.be/Crj0uYcil-8. Accessed 28 Sept. 2023.