Wildfire Mapping Using SAR-Derived NBR through Generative Adversarial Network
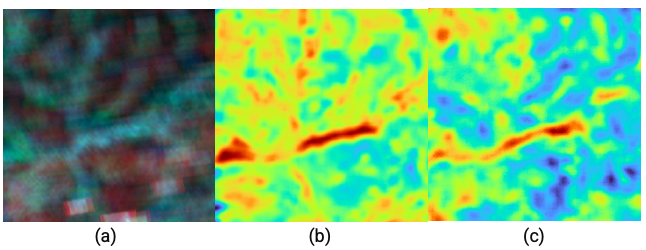
10 April 2024
Donato Amitrano, PhD in Remote Sensing, Co-Founder and R&D Manager at Latitudo 40
Introduction
Wildfires have become increasingly prevalent, fueled by climate change and its associated effects, such as higher global temperatures and extended periods of drought. Nonetheless, fires with elevated severity levels, inflicting widespread devastation, have been increasingly reported across regions such as the United States, the Mediterranean basin, and Australia, raising concerns over the efficacy of prevailing management strategies. These high-severity wildfires result in significant landscape alteration and degradation, challenging the sustainability of affected ecosystems.
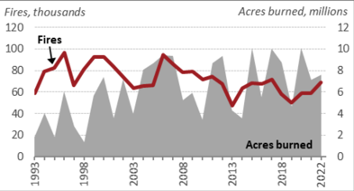
Figure 1. Annual Wildfires and Acres Burned, USA, 1993-2022. Source: NICC Wildland Fire Summary and Statistics annual reports
Though fluctuating, the annual extent of land impacted by wildfires has shown a general uptrend (see Figure 1). In USA, since the year 2000, there has been an average of 70,025 wildfires each year, consuming around 7.0 million acres. This area is over twice the size of the land affected on average annually during the 1990s, which was approximately 3.3 million acres, despite the 1990s experiencing a higher average annual number of fires, estimated at 78,600.
The delineation of large-scale fire severity, particularly within topographically complex terrains, poses challenges for conventional field-based methodologies. In this context, remote sensing technologies offer a valuable tool for evaluating wildfire impacts. Advancements in satellite technology, enhancing spatial resolution capabilities, have facilitated the precise determination of the spatial extent of burn scars, the ongoing monitoring of wildfire progression, and the evaluation of fire severity.
Using Sentinel-2 satellite imagery, characterised by its 10-m spatial resolution, alongside spectral indices, has been integral to fire severity assessments. Among these indices, the Normalised Burn Ratio (NBR) and the Normalised Difference Vegetation Index (NDVI) are prevalently employed, with empirical evidence suggesting a higher sensitivity of NBR to the spectral variations induced by moderate to high-severity fires. Despite the efficacy of optical imagery in monitoring and evaluating fire severity, limitations persist, including obscuration by cloud cover, smoke, and haze, which compromise the effectiveness of visible and infrared spectrum-based sensors.
In scenarios where optical imagery is rendered ineffective due to atmospheric obstructions, Synthetic Aperture Radar (SAR) images from the Sentinel-1 mission emerge as a viable alternative or supplementary resource, enriching the data available for surface analysis. SAR-based burned area mapping leverages the variability in radar signal backscattering contingent on fire severity. Empirical investigations have demonstrated the responsiveness of VH polarisation to burn events.
In the context of wildfires, sensors with shorter wavelengths typically observe a reduction in backscattered energy following the canopy's removal. This decrease is primarily due to the elimination of volumetric scattering provided by leaves (Ban et al., 2020) [1]. Nonetheless, this observed pattern may not always hold true due to two key factors: firstly, the revelation of soil layers previously obscured by vegetation alters the radar's sensitivity to changes in the soil's dielectric properties, which can vary with moisture levels (Ban et al., 2020); and secondly, the absence of leaves may enhance the occurrence of double-bounce scattering effects, stemming from the remaining branches (Bourgeau-Chavez et al., 1997) [2]. These factors can contribute to an unexpected increase in the energy backscattered to the sensor.
Methodology
Given radar signals' complex and sometimes unpredictable response in analysing forest fires, an approach has been experimented with to derive NBR from SAR images (Figure 2). The process begins with collecting SAR images over time from the Sentinel-1 satellite. Multi-temporal SAR pre-processing accounts for radiometric calibration, geometric co-registration, multi-temporal despeckling, time-series cross-calibration, multi-temporal composites generation and geocoding.
The processed SAR images are inputted into a Generative Adversarial Network (GAN). The output from the GAN, which now consists of optical-like images, is then used to calculate the Normalised Burn Ratio (NBR). GANs use two neural networks competing against each other to improve the quality of image generation or translation. In this context, the GAN converts the SAR images into NBR maps. The generator creates images that resemble NBR maps while the discriminator evaluates them against actual NBR maps derived from optical data, guiding the generator to produce increasingly accurate translations.

Figure 2. Workflow of the proposed approach.
The aim is to map the area affected by wildfire using NBR comparison between pre- and post-events: the synthetic post-event NBR is combined with real multispectral pre-event NBR to form the difference used to classify burned areas.
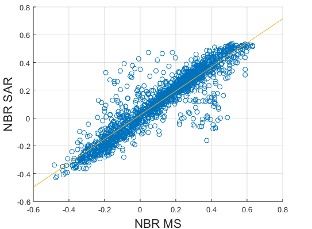
Figure 3. Scatter plot between native NBR from Optical Multispectral (MS) Sentinel-2 images and NBR derived from SAR Sentinel-1 with the proposed approach.
Figure 3 shows the scatterplot between native NBR from Optical Multispectral (MS) Sentinel-2 images and NBR derived from SAR Sentinel-1 with the proposed approach. Results were computed on 1700 patches, obtaining an RMSE of 0.140 and an R2 equal to 0.850.
Figure 4 shows the multitemporal SAR image input, the NBR derived from the Sentinel-2 multispectral image, and the NBR computed using the proposed GAN methodology. This shows the practical application and congruence of the derived data.
Figure 4. (a) Multitemporal SAR Sentinel-1 image input; (b) NBR derived from Sentinel-2 Multispectral Image; (c) NBR computed with the proposed approach
Conclusion
This study demonstrated the efficacy of a novel approach to wildfire severity mapping by integrating SAR imagery and Generative Adversarial Networks (GANs). By employing a method that processes SAR data from the Sentinel-1 satellite to simulate optical-like images, this approach advances the capability to generate Normalised Burn Ratio (NBR) indices that are traditionally derived from optical multispectral (MS) imagery.
The results underscore the potential of SAR data, when combined with advanced computational techniques such as GANs, to overcome the limitations posed by atmospheric obstructions in optical imagery. The accuracy of the GAN-derived NBR maps promises to enhance the monitoring and management of wildfire-affected areas by providing reliable data essential for informed decision-making. However, the study acknowledges the need for further validation across different ecosystems and fire conditions to universalize the applicability of this methodology.
In the context of the TEMA project, the promising outcomes pave the way for improved wildfire assessment, enabling more precise mapping and analysis that can support the development of effective fire management strategies and contribute to the conservation of ecosystems under the increasing threat of severe wildfires.
References:
[1] https://www.nature.com/articles/s41598-019-56967-x
[2] https://www.tandfonline.com/doi/abs/10.1080/014311697219114