Using Diffusion Models to Generate Datasets of Images Affected by Natural Disasters
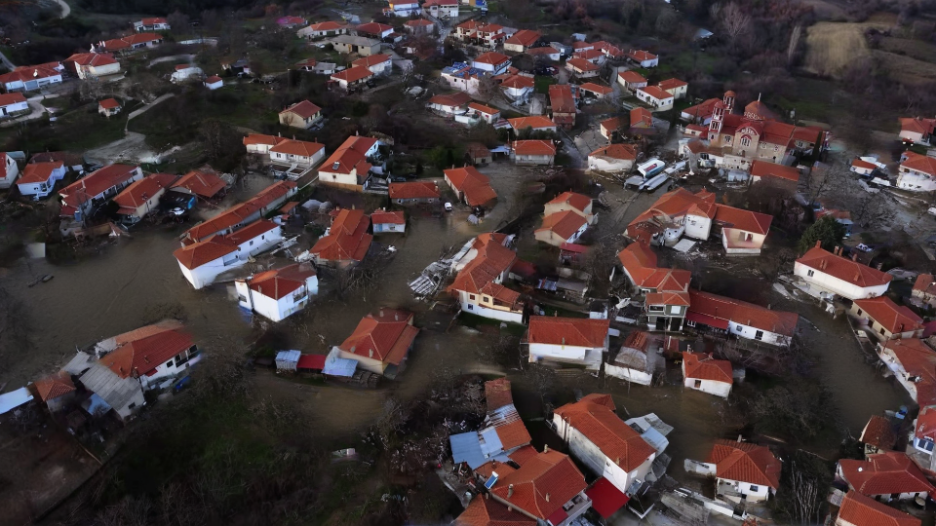
11 December 2024
Eleonor Diaz, Computer Vision Engineer at Atos Spain
In recent years, diffusion models have become powerful tools in the field of artificial intelligence for image generation. Their applications extend beyond traditional creative or artistic uses, proving highly valuable for scientific, engineering, and crisis management purposes. One particularly innovative use of diffusion models is in creating datasets that simulate the effects of natural disasters, such as floods, wildfires, earthquakes, or hurricanes, on locations that are typically undamaged. This capability has immense potential in areas like emergency preparedness, environmental monitoring, and urban planning.
A major advantage of using diffusion models for disaster image generation is their ability to produce realistic images across a wide range of scenarios. These models achieve this by learning patterns from existing data. The resulting images can depict infrastructure collapse, floods, fires, or debris accumulation in a way that is visually convincing, providing valuable datasets that closely mirror real-world disaster scenarios.
Beyond producing realistic images, diffusion models play a crucial role in enhancing disaster preparedness and risk assessment. Governments, urban planners, and emergency response teams depend on realistic disaster simulations to predict future events and mitigate potential risks. By generating disaster-affected images from undamaged locations, diffusion models allow decision-makers to visually assess specific risks in different areas. For instance, simulations showing rising sea levels or storm surges in coastal cities help inform flood defense strategies and emergency planning.
Another significant benefit of diffusion models is their ability to generate datasets at scale. In disaster-related AI research, one of the biggest challenges is the scarcity of large, diverse datasets representing different types of disasters across various landscapes. Collecting real-world images from disaster-stricken areas is often time-consuming and constrained by geographic and temporal limitations. Diffusion models address this issue by enabling the generation of virtually limitless disaster-affected images from a small set of undamaged ones. This scalability is particularly valuable for training robust machine learning models that require extensive data for accurate predictions.
Furthermore, using diffusion models in this context is both cost-effective and safe. Collecting real-life images from disaster-hit regions can be expensive, hazardous, and logistically difficult. By generating disaster-affected datasets with diffusion models, researchers and organizations can acquire high-quality data without exposing anyone to dangerous environments or incurring the high costs associated with field data collection. This approach is especially beneficial for rare or unpredictable events, where gathering timely real-world data may not be possible.
In our work, we have incorporated drone technology to capture high-resolution aerial images of undamaged locations, which are then fed into diffusion models to simulate flood effects. The models allow us to realistically introduce flood conditions into these images, simulating rising water levels, debris accumulation, and water reflections. This method enables us to visualize potential flood scenarios in areas that have yet to experience such events, providing valuable insights for disaster preparedness, particularly in urban environments where infrastructure vulnerabilities are critical.
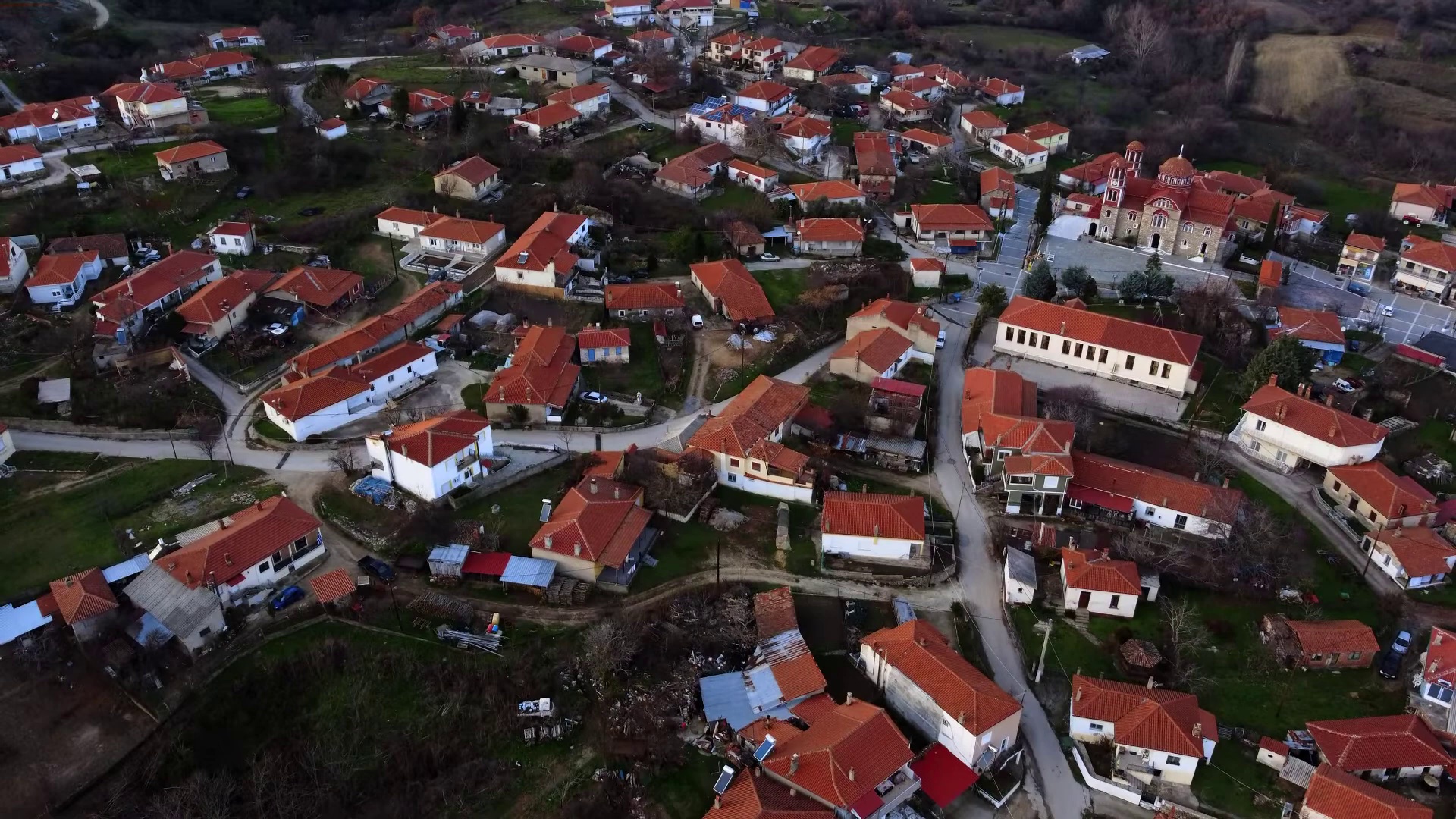
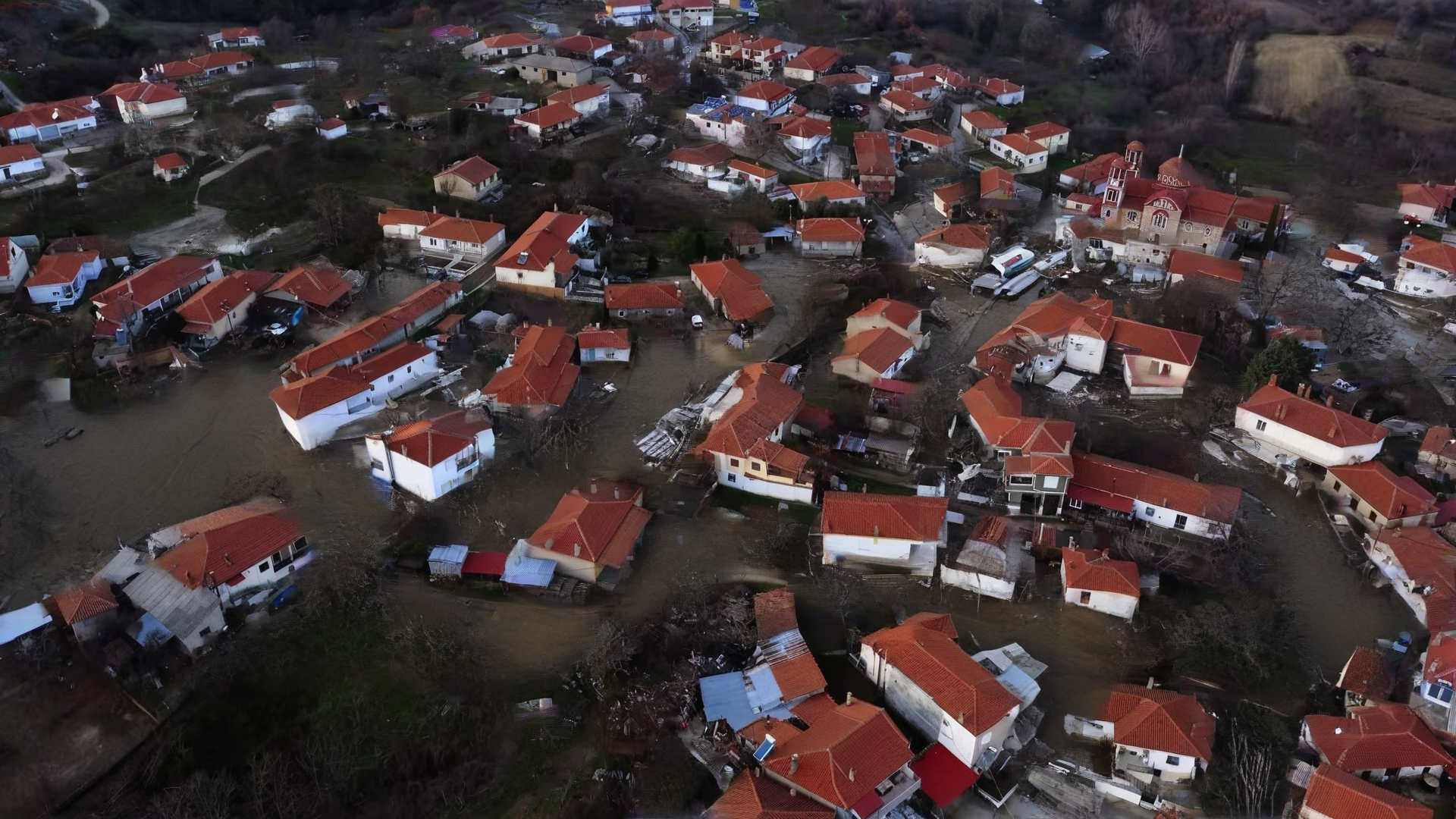
Ethical considerations are also an important aspect of using diffusion models for disaster image generation. In some cases, showing real images of disaster-affected areas may be distressing to the public or raise privacy concerns. Synthetic images generated by diffusion models avoid these issues, as they are based on simulations rather than real-world suffering or destruction. Additionally, diffusion models enable controlled experimentation, allowing researchers to explore hypothetical disaster scenarios with different intensity levels.
Lastly, diffusion models can significantly enhance AI systems used in post-disaster relief efforts. AI models increasingly rely on satellite and aerial images to assess damage following natural disasters, helping coordinate relief and recovery operations. These models, however, often require large amounts of labeled data to accurately identify and classify damage. Diffusion models can augment existing datasets by generating high-quality disaster images, enabling AI systems to improve their performance in damage classification, prioritization of rescue efforts, and resource allocation.
In conclusion, diffusion models are invaluable for generating realistic, scalable, and customizable images of natural disasters from undamaged locations. By integrating drone technology and applying diffusion models to simulate flood scenarios, we can create data-driven images that enhance disaster planning and response strategies. These models provide an ethical, cost-effective, and risk-free alternative to traditional data collection, contributing to more effective responses to natural disasters.
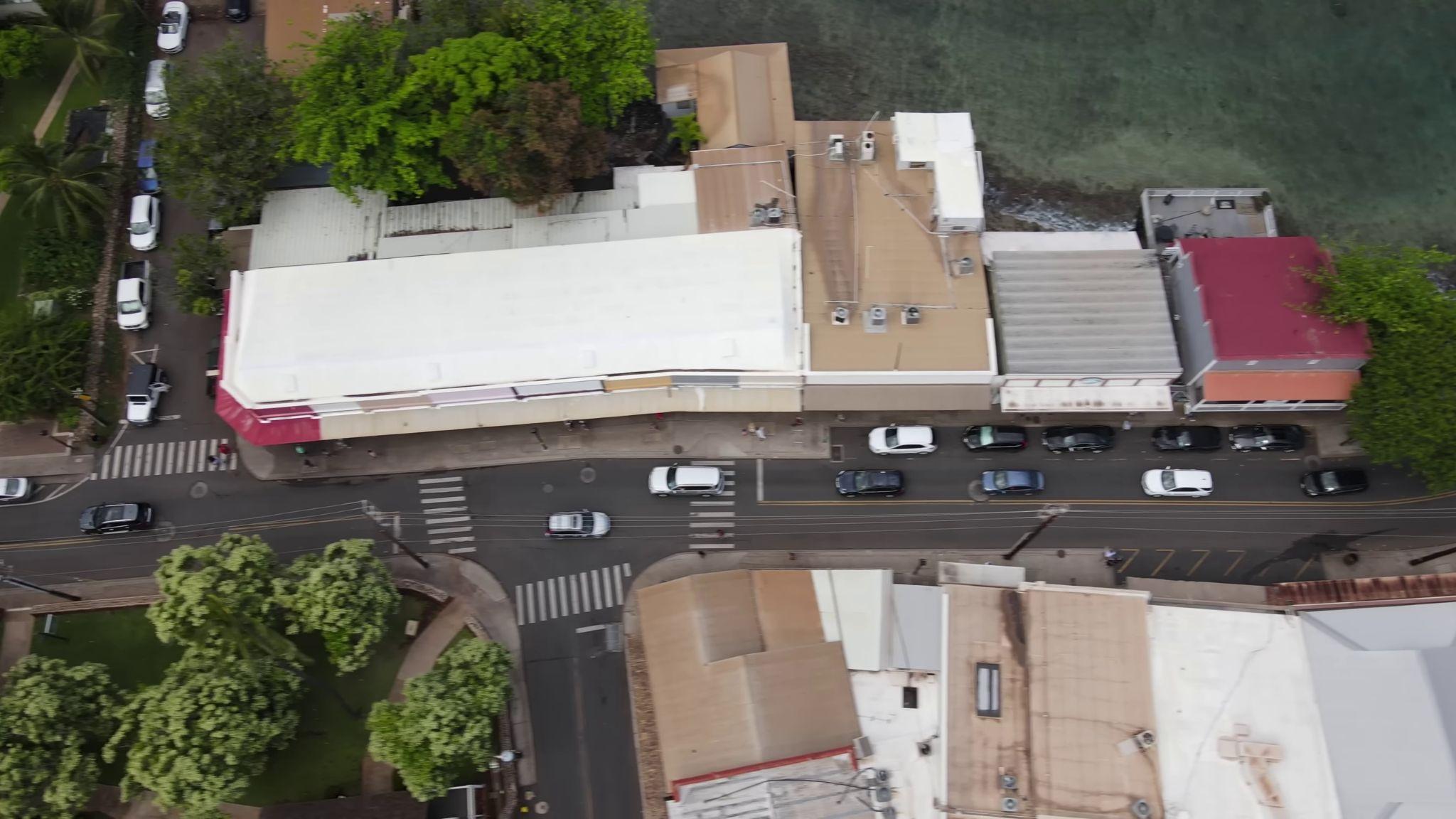
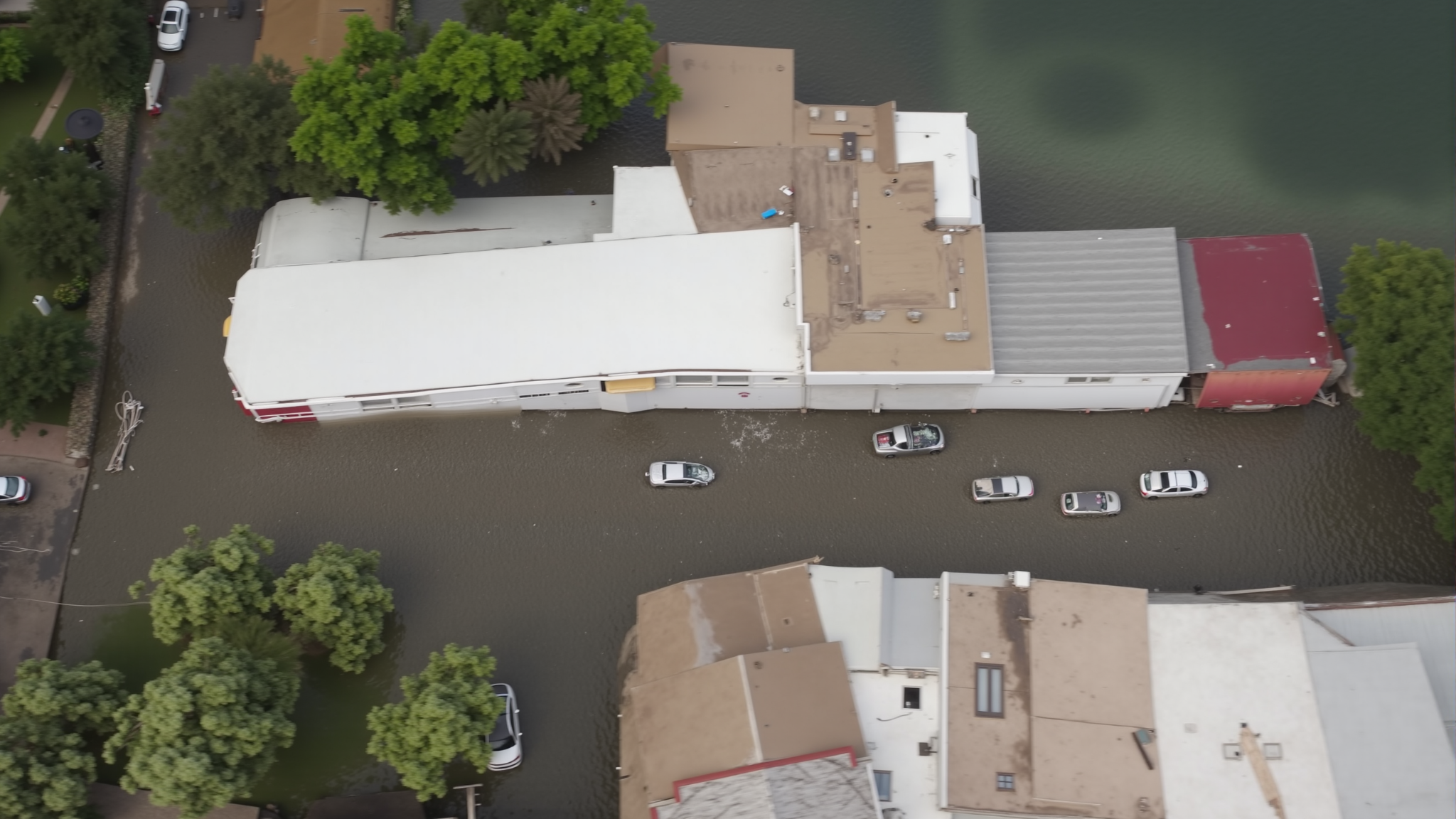