Integrating Advanced Weather Data with Utility Asset Outage Analysis to Quantify Wildfire Risk
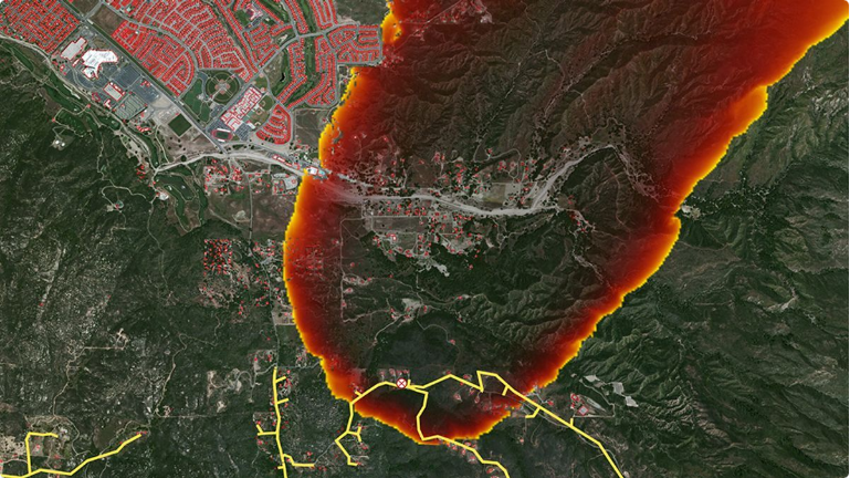
23 July 2023
Pavel Grechanuk, Senior Wildfire Risk Data Scientist at Tecnosylva
Energy utilities across the United States continue to face the challenge of reducing wildfire risk while also increasing their reliability as part of their ongoing operations. As droughts and weather events accelerate the spread of wildfire to more and more communities, we are seeing that this risk is not just a “California issue” anymore. Not only do risk managers at energy utilities need to know the probability of a wildfire, but they also need to know the consequence to their assets and service area from one ignition compared to another. In short, not all ignitions (fires) are created equal. Risk managers need the tools to understand the likelihood of a utility-cased ignition as they balance their overall wildfire risk.
Leveraging high-resolution weather dataset forecasts over your entire service territory and then simulating fire ignitions across all your assets allows you to tie results of those simulations back to the asset itself. Yet, how likely are those consequences to materialize? Here, you need dynamic probability outage, failure and ignition models in order to quantify the likelihood of a specific asset starting a specific wildfire under a range of possible weather conditions.
To achieve this, we look at two components of outage analytics. The first is probability of failure, which is a failure along a line segment that results in a spark or burning material on the ground. This can relate to a knockdown or vegetation contact as well. The second component is the probability of ignition, which is the probability that burning material – the coupling of wind, temperature, and vegetation information – will create a wildfire that needs suppression. If you combine probability of failure with the probability of ignition you can determine the likelihood of a specific asset starting a wildfire.
What does this likelihood tell you about your risk, though? Here, we are presented with our conditional risk, which can be captured by simulated impacts of a virtual wildfire occurring at a specific point of location, time, and weather condition. This illustrates how many acres a possible fire is going to burn unsuppressed and how many structures and assets it can possibly impact. What you don’t know is if that fire could actually occur in the first place. This is where expected risk comes into play. Expected risk is the combination of probability of failure, probability of ignition, and those simulated wildfires impacts across the landscape. With these key factors in play, you can now see if a specific asset can ignite a consequential wildfire and plan accordingly.
This is where modeling weather comes into play, especially wind severity. Looking at a specific substation, you can compare the total number of outages to a range of wind speeds. Initially, results will illustrate that your highest number of outages are at lower wind speeds, which seems incorrect. This is because you’re considering the sum (total), instead of the average (balance of spread) at each wind speed. Exploring the percentage of time there's an outage at each of these incidents makes the relationship much clearer about why wind is such an important factor in outages. Here, the largest percentage of outages for a given asset will occur at a higher speed. Thus, every single circuit and every single substation has a unique fragility curve for each asset.
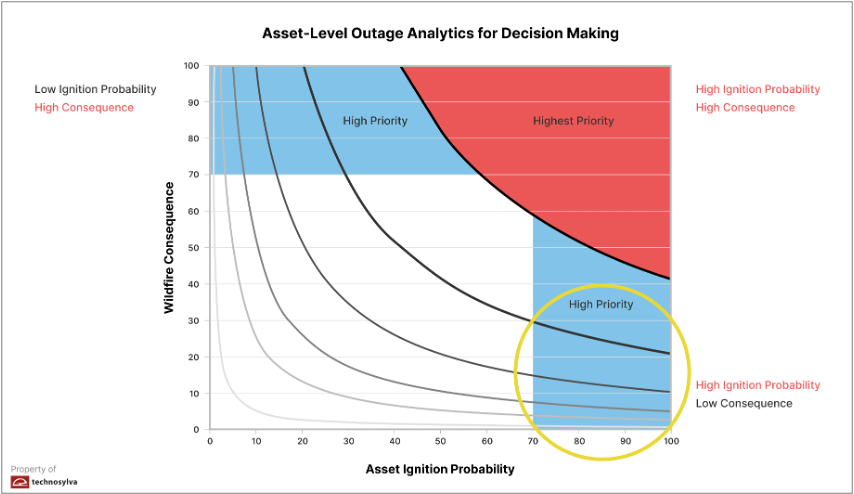
So, what does this give you for operational and mitigation decision-making? First, it helps you to identify long term system hardening planning priorities. If you can identify your most sensitive circuits to wind, you can assess expected impacts, like vegetation contact, equipment failure, or fire severity potential. Driving this curve to the right helps to define certain steps you can take that are most optimal for your invested resources. This information also helps in staging personnel during upcoming windstorms, so instead of forecasting wind gusts, you can start forecasting possible ignitions. If you can identify where you’re most likely to have outages, you can get out ahead of your wildfire risk.
In TEMA project, the weather data assimilation model used by the Wildfire Analyst® FireSim simulator will be improved, contributing to generate better fire risk predictions and assisting in better decision making and management of natural disasters caused by fire.