Geo-social Media Data Analysis for Triggering Satellite-Based Emergency Mapping
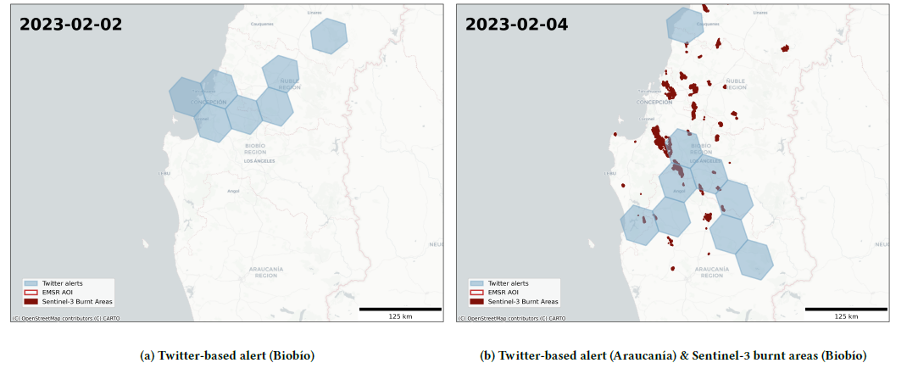
13 November 2023
Bernd Resch, Associate Professor and Head of “Geo-social Analytics Lab” at PLUS;
Sebastian Schmidt: Researcher and PhD student at PLUS.
When a natural disaster occurs, it is particularly important to obtain information about which regions are affected as quickly as possible. Remote sensing data, especially from earth observation satellites, is often used to analyse the course of natural disasters. However, most satellites are on fixed orbits or have to be explicitly tasked in order to take images of a certain region. This always results in a time delay.
Significant progress has already been made in the area of data analysis in recent years, which has led to a speeding up of the process. However, the activation of satellite-based emergency mapping (SEM) is still a manual action by an authorised user. The identification of an area of interest (AOI) for explicit tasking, i.e. controlling the satellite for image acquisition, is also necessary in advance.
A collaboration between the University of Salzburg and the German Aerospace Centre (DLR) investigated the extent to which data from geo-social media could speed up this process. Data from social media has already been described in many studies as a useful and important data source in the disaster management process. It allows a wide range of information to be extracted in near-real time, which can represent both objective and subjective descriptions of the situations. Image content in particular can also offer great added value for first responders.
To this end, a model was developed that can recognise whether a post (e.g. a Tweet) refers to a natural disaster or not. It is built on state-of-the-art natural language processing methods, namely the Twitter-XLM-RoBERTa-base model. The former is a robust variant of the popular transformer-based BERT architecture, which is able to analyse texts in their context. This model was finetuned with large training datasets from CrisisLex. In addition, an active learning approach was then pursued, whereby further data was annotated by experts, thus iteratively increasing the model quality. The final model achieved an accuracy of 0.97 on the CrisisLex data and an accuracy of 0.90 on a specific test data set of the case study.
An important part of the study was the spatial analysis. For this purpose, the Tweets, which all had a georeference (usually polygons that roughly represent the region in which the Tweet was presumably sent), were aggregated onto a hexagonal grid. Based on the proportion of relevant Tweets per grid cell, alerts were then automatically generated that marked the start of a disaster. For this purpose, a deviation of the ratio from a baseline from the previous year was determined.
The severe forest fires that raged in southern Chile in early 2023 were chosen as the use case for an initial case study. The regions of Ñuble, Biobío and La Araucanía were particularly badly affected at the beginning of February. The triggers were a prolonged drought affecting large parts of the country and a severe summer heatwave. However, it is also assumed that many of the fires were caused by arson[1]. In order to analyse this event, the scientists collected geo-referenced data from the social media platform Twitter via the official APIs. The model described above was then used to classify these Tweets and generate the alerts with a spatial reference.
Using their methodology, the researchers were able to identify potentially affected areas earlier than with official disaster alerts. In future, satellite tasking and thus data acquisition in natural disasters could therefore begin earlier. Consequently, the outputs of this study are of high importance for the TEMA project, where both earth-observation data and information from geo-social media are central inputs. The methodology developed here could therefore also be used to speed up the data acquisition process for use cases within TEMA and hence improve the disaster management process, which is the main project objective. The collaboration between the University of Salzburg and the German Aerospace Center (DLR) needed for this study was only made possible by the TEMA project.
Figure 1 Comparison of disaster alerts
So far, the proposed methodology has only been validated on the basis of one use case. Future analyses must therefore examine the transferability to other types of natural disasters (e.g. floods) and other geographical areas. Particularly in the European context, where the Global Disaster Alert and Coordination System (GDACS) and European Flood Awareness System (EFAS) are more complex or more reliable warning systems, the added value of social media data for early warning could be lower. A more complex method for alert generation that can explicitly include space in the calculation is also being considered. For example, spatial hot spot analyses could be included here.
The study described above was based exclusively on data from Twitter (now X). Due to internal reorganisation, data availability for research is no longer guaranteed at the moment. For this reason, the transferability of the methods developed to data from other networks (e.g. Facebook, Telegram, YouTube) should be reviewed.
A corresponding paper by Sebastian Schmidt, David Hanny, Monika Friedemann, Bernd Resch, Torsten Riedlinger and Martin Mühlbauer is currently in the works.
If you have any questions, please contact either sebastian.schmidt@plus.ac.at or bernd.resch@plus.ac.at
For updates on our research, follow us on LinkedIn (www.linkedin.com/company/geo-social-analytics-lab) or check out our website (https://geosocial.at/).
Bibliography:
[1] https://lahora.cl/cronica/2023/02/04/corma-acuso-intencionalidad-tras-incendios-forestales-se-han-aportado-pruebas-a-fiscalia/