Fire behaviour modelling for operational decision-making
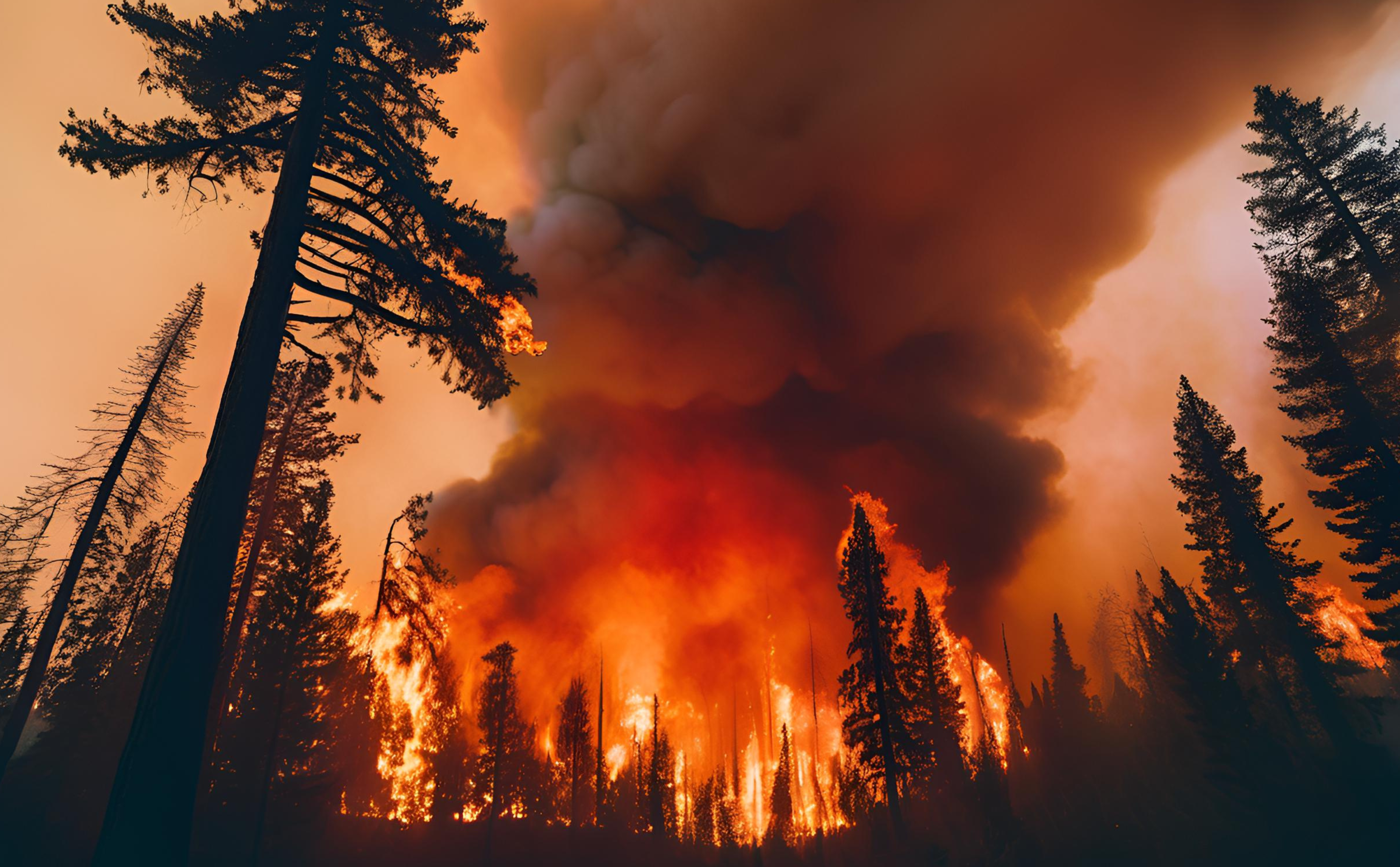
26 November 2024
Adrián Cardil Forradellas, PhD., Senior Fire Modelling Scientist at Tecnosylva.
Wildfire is an integral component of many ecosystems, often necessary for habitat renewal and biodiversity. However, as recent events have shown, wildfires can also inflict severe damage and impacts on communities, infrastructure and the environment. Wildfires pose a growing threat globally, with their frequency and intensity exacerbated by climate change, urbanisation and changes in land use. Predicting fire behaviour is critical for ensuring safety, optimising resource deployment and mitigating damage. This article outlines the progress in modelling technologies, the associated challenges and the evolving applications in operational contexts.
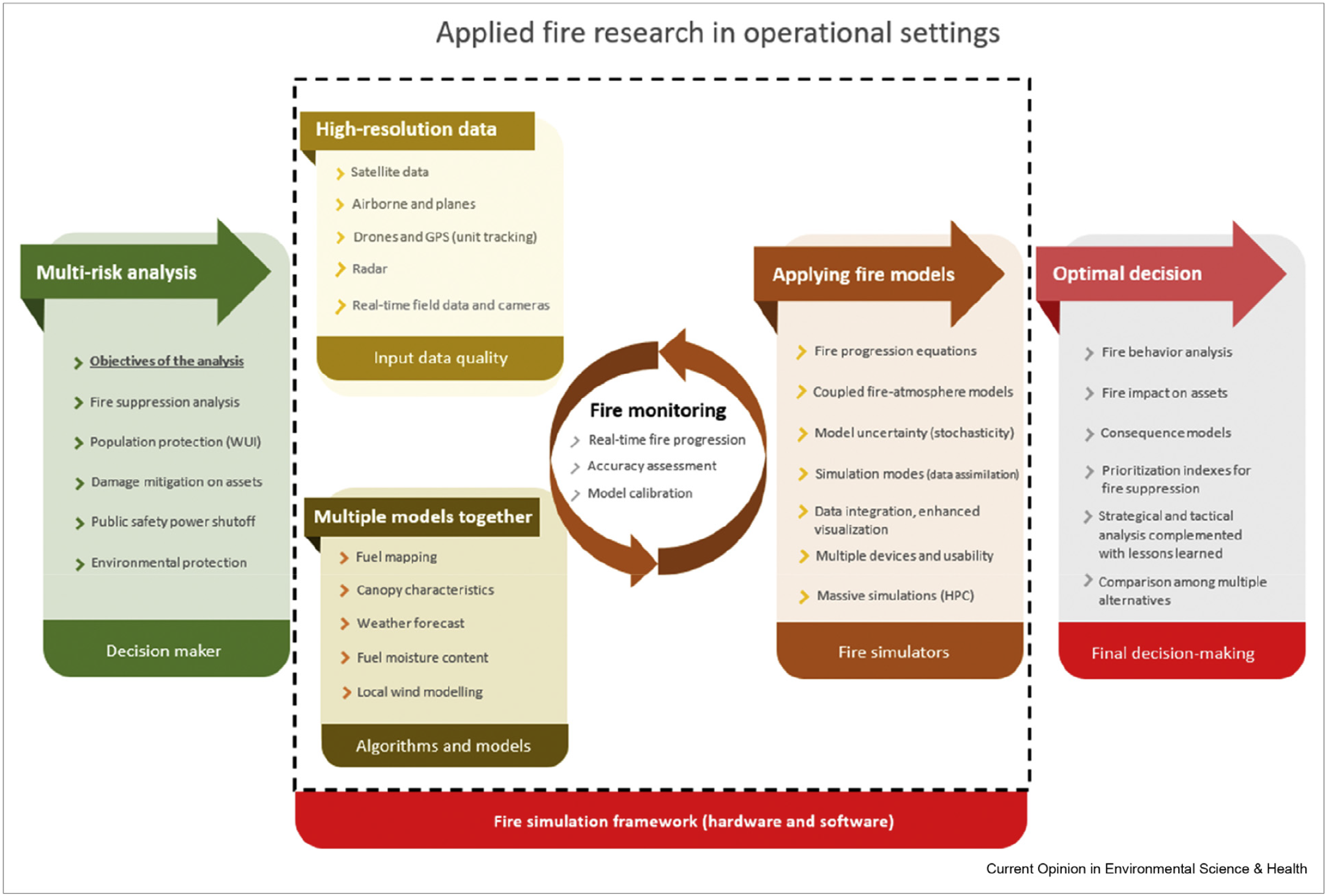
Importance of Fire Behaviour Modelling
Wildfire behaviour models simulate the dynamics of fire spread, intensity and impact by integrating various environmental variables, such as:
- Weather Conditions: Wind speed, humidity and temperature directly influence fire intensity and spread rate.
- Topography: Slope and elevation alter fire dynamics by accelerating spread or hindering growth in different directions.
- Fuel Characteristics: The type, density and distribution of vegetation (fuel model) significantly determine the energy available for combustion.
Accurate modelling aids in resource allocation, evacuation planning and containment strategy formulation. However, achieving precision in these models is challenging due to the complex interactions among the variables.
Advancements in Fire Behaviour Models
Below is a summary of the progress that has been made in fire behaviour modelling:
Data Integration and Remote Sensing
Modern fire models benefit from advances in spatial data acquisition, such as satellite imaging, LiDAR and unmanned aerial vehicles (UAVs). Technologies like NASA's GEDI LiDAR system offer detailed 3D measurements of forest structures, providing insights into canopy fuel loads and bulk density. Additionally, the fusion of spectral and LiDAR data enhances the identification and mapping of fuel types based on their optical and structural properties. These methods improve predictions and allow faster updates, compared to traditional field surveys.
Coupled Fire-Weather Models
Fire behaviour is deeply intertwined with atmospheric conditions. Coupled fire-weather models integrate predictions of fire dynamics with near-surface weather forecasts to account for real-time feedback loops, such as how fire-generated heat and winds alter atmospheric patterns. These interactions, often referred to as fire-atmosphere coupling, are critical for understanding rapid fire growth and extreme fire phenomena. While these models have shown promise, their operational deployment is hindered by computational complexity, resource demands and the need for specialized expertise.
Data Assimilation and Optimisation Techniques
Real-time observations of active fires, such as satellite hotspot data or ground temperature readings, can be assimilated into running simulations to adjust parameters and improve accuracy. Tools like Kalman filters dynamically update the fire perimeter and factors such as fuel adjustment rates, while particle filters or genetic algorithms optimize environmental variables (e.g., canopy cover). Despite these innovations, many algorithms remain computationally intensive, limiting their use in urgent operational contexts. Emerging methods like Derivative-Free Optimization or gradient-based techniques aim to address these challenges by offering faster, more efficient solutions.
Simulation Modes
Fire behaviour models are employed in different simulation modes to address diverse operational needs:
- Predictive Mode: Models forecast fire spread and intensity, informing containment strategies and resource allocation.
- Inverse Mode: By reconstructing the fire’s origin and trajectory, these simulations identify likely ignition points, useful for forensic investigations or validating containment success.
- Asset-Centric Analysis: Simulations prioritize protecting critical assets or populations, such as defining evacuation perimeters or planning firefighter escape routes. This involves calculating the time a fire might take to reach specific locations and implementing proactive defences.
Challenges in Fire Behaviour Modelling
The challenges currently facing the modelling of fire behaviour include, among others:
Extreme Fire Events
Extreme fire behaviour, characterised by rapid spread, erratic movements and phenomena like pyrocumulonimbus (fire-induced thunderstorms), poses significant modelling challenges. These fires often influence their surrounding environment, creating feedback loops that conventional models struggle to predict. Pyroconvective activities, including wind surges and fire-generated lightning, further complicate the reliability of weather and fire predictions. As the frequency of such events increases due to climate change, models must evolve to account for these dynamics.
Expanding Fire-Prone Regions
Climate change has altered the distribution of wildfire activity, with traditionally temperate or wet regions experiencing unprecedented fire events. The shifting climate zones introduce new challenges, as models must adapt to unfamiliar vegetation types, weather conditions and fire behaviours. For example, regions in northern Europe or boreal forests, once relatively fire-resistant, now experience increased risks.
Operational Constraints
Advanced fire modelling systems, while effective, often require substantial computational power, technical expertise and integration with other systems, such as emergency response frameworks. These demands limit their widespread adoption in operational contexts. There is a pressing need for user-friendly, efficient models that balance accuracy with speed for real-time applications.
Emerging Trends and Innovations
To meet the challenges posed by extreme fire events and new fire-prone regions, fire behaviour modelling is evolving in several key directions:
Improved Data Sources
Enhanced satellite systems and UAV technology allow for greater temporal and spatial resolution in monitoring active fires and fuel conditions. For example, multispectral imaging captures vegetation health and moisture content, while UAVs provide close-range views of fire perimeters in real time.
Artificial Intelligence and Machine Learning
AI techniques are increasingly used to analyse historical fire data, identify patterns and predict future fire behaviours. Machine learning models can integrate vast datasets, including weather forecasts and vegetation properties, to refine predictions dynamically.
Simplified and Accessible Models
Efforts are underway to develop models that are computationally lightweight yet retain high accuracy. These tools aim to empower fire managers and first responders with actionable insights without requiring advanced technical skills or extensive computational resources.
Holistic Simulation Approaches
Combining predictive, inverse and asset-centric simulation modes within a unified platform enables comprehensive wildfire management strategies. For instance, integrated systems can simultaneously identify high-risk areas, simulate evacuation scenarios and assess containment success probabilities.
Collaboration and Knowledge Sharing
Partnerships between researchers, emergency responders and policymakers are essential for translating theoretical advancements into practical solutions. Sharing data, experiences and methodologies across regions accelerates the refinement and adoption of fire behaviour models globally.
Broader Implications and Future Directions
Fire behaviour modelling plays a critical role in addressing the increasing severity and complexity of wildfires worldwide. Beyond operational decision-making, these models contribute to broader environmental and societal goals:
- Risk Mitigation: By identifying high-risk regions and simulating potential fire scenarios, models support proactive measures like controlled burns or fuel reduction programs.
- Climate Adaptation: Modelling informs climate adaptation strategies by projecting future wildfire risks under various scenarios, guiding resource allocation and policy decisions.
- Community Resilience: Improved predictions enable better evacuation planning and infrastructure design, enhancing community safety during fire emergencies.
This study underscores the necessity of continuous innovation in fire modelling to stay ahead of the escalating wildfire challenges. The TEMA project is making it possible by improving Tecnosylva's FireSim (Wildfire Analyst) simulation models by
- Enhancing its weather forecast model by ingesting real-time weather data measured in the field to adapt the weather forecast model to the real weather conditions in the fire area.
- Adjusting its simulations results with information on the position of fire fronts at any given time, so simulation parameters are modified depending on fuel model and fire line position.