EmoGRACE: Advancing Emotion Analysis for Social Media Data
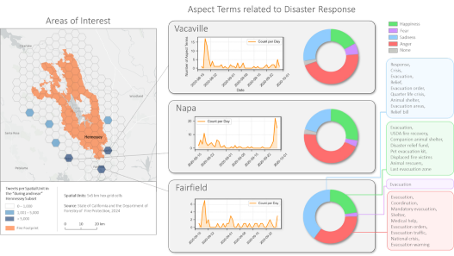
7 May 2025
Sebastian Schmidt, Researcher at Geo-social Analytics Lab / Department of Geoinformatics at PLUS
Shaily Gandhi, Senior Postdoctoral Fellow at IT:U
In the discipline of Natural Language Processing (NLP), sentiment analysis has evolved from basic polarity classification (positive vs. negative) of entire texts or sentences to more nuanced aspect-based sentiment analysis (ABSA), which identifies sentiments related to specific terms or phrases within a text. However, the equivalent progression in emotion analysis has been hindered by the complexity of emotions and a lack of annotated datasets.
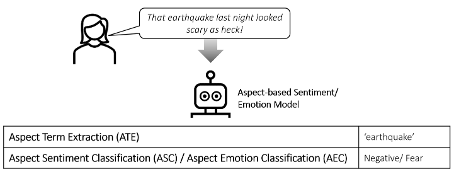
Addressing this gap, researchers from the University of Salzburg have introduced EmoGRACE, a pioneering approach to Aspect-Based Emotion Analysis (ABEA) tailored for the more fine-grained analysis of social media data.
Building the Foundation: A New Dataset
Recognising the scarcity of ABEA datasets, a novel dataset comprising 2,621 English Tweets was created and manually labelled. The annotation process was grounded in Shaver et al.'s (1987) hierarchical emotion theory, categorising emotions into Anger, Sadness, Happiness, Fear, and a 'None' class. To ensure consistency, a group annotation strategy with majority voting was employed.
Leveraging GRACE for Emotion Analysis
The researchers fine-tuned the existing GRACE model by Luo et al. (2020) - originally designed for ABSA - for the tasks of Aspect Term Extraction (ATE) and Aspect Emotion Classification (AEC). Model performance plateaued at an F1-score of 70.1% for ATE and 46.9% for the combined ATE and AEC tasks. This limitation was attributed to the training dataset's limited size and the inherent complexity of emotion classification.
Application for disaster management
As a proof of concept, the EmoGRACE framework was applied to a disaster scenario -analysing geo-tagged Tweets related to the 2020 Californian wildfires. This case study demonstrated the model’s potential in capturing nuanced emotional dynamics over space and time. Emotional patterns were found to cluster geographically, with Tweets from regions closer to wildfire perimeters exhibiting heightened levels of sadness, fear, and anger. Temporally, emotional responses surged during major fire events, often aligning with critical developments such as evacuations or containment updates. Interestingly, moments of collective relief, such as successful firefighting efforts, saw brief upticks in expressions of happiness. These findings highlight how spatio-temporal ABEA can offer valuable insights into public sentiment during crises, emphasising the importance of continued research in this intersection of emotion analysis and disaster response.
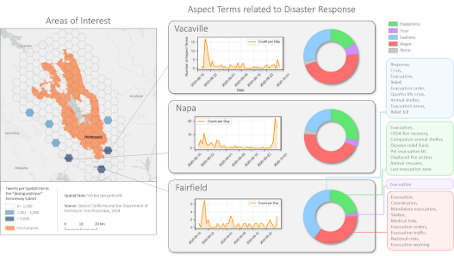
Conclusion
EmoGRACE represents a significant step toward nuanced emotion analysis in social media, providing a foundation for future advancements in ABEA. By introducing a new dataset and adapting existing models, this work paves the way for more emotionally intelligent NLP applications. Future research directions include expanding the dataset, exploring multilingual capabilities, and integrating more sophisticated models to enhance performance.
For a detailed exploration, please refer to the full discussion paper: EmoGRACE: Aspect-based emotion analysis for social media data.
Bibliography
Luo, H., Ji, L., Li, T., Jiang, D., & Duan, N. (2020). GRACE: Gradient Harmonized and Cascaded Labeling for Aspect-based Sentiment Analysis. Findings of the Association for Computational Linguistics: EMNLP 2020, 54–64. https://doi.org/10.18653/v1/2020.findings-emnlp.6
Shaver, P., Schwartz, J., Kirson, D., & O’Connor, C. (1987). Emotion knowledge: Further exploration of a prototype approach. Journal of Personality and Social Psychology, 52(6), 1061–1086. https://doi.org/10.1037/0022-3514.52.6.1061
Zorenböhmer, C., Schmidt, S., & Resch, B. (2025). EmoGRACE: Aspect-based emotion analysis for social media data (Version 1). arXiv. https://doi.org/10.48550/ARXIV.2503.15133