Drowning in the information flood: Identifying relevant social media posts for disaster management
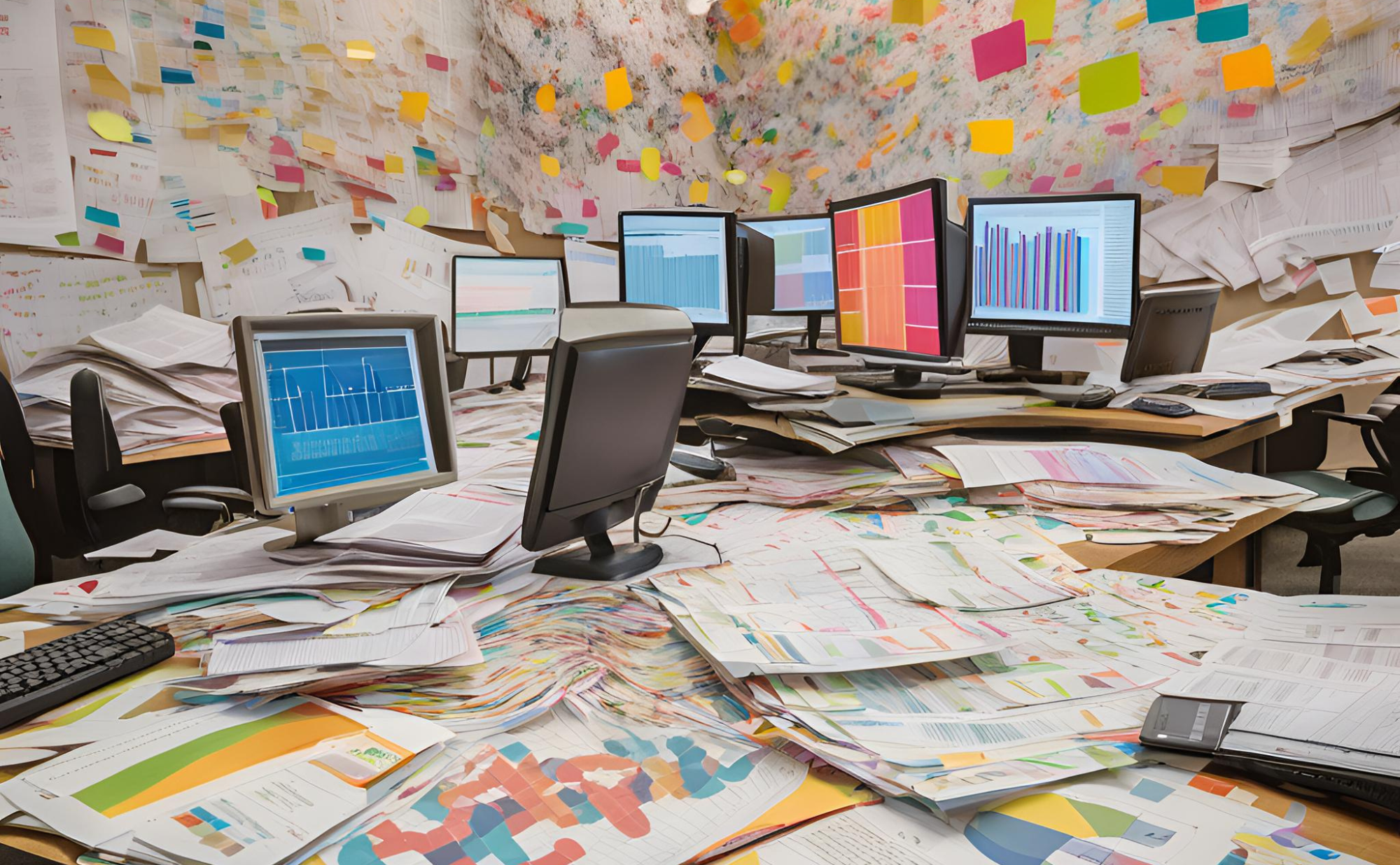
5 December 2024
Sebastian Schmidt, Researcher at Geo-social Analytics Lab / Department of Geoinformatics at PLUS
Bernd Resch, Associate Professor & Head of Geo-social Analytics Lab / Department of Geoinformatics at PLUS
Data from geo-social media can provide great added value for disaster management, as they offer a wide range of information (texts, images, videos, etc.) in near-real time. However, the large amount of data produced by users in the process poses major problems for emergency responders: Which posts contain information that offers added value for an operation? How can this information be filtered to save as much time as possible?
In order to be able to offer such an automated workflow, researchers at the University of Salzburg developed a methodology for relevance classification of Tweets for disaster management. A big part of the work was the theoretical definition of "relevance": what kind of Tweet can really add value to a first-aid organisation? What content is particularly relevant? To clarify these questions and subsequently fine-tune machine learning models based on these agreements, close collaboration was conducted with TEMA experts from the Red Cross and external partners from THW (German Federal Agency for Technical Relief) and Johanniter.
To identify potentially relevant Tweets, different machine learning methods (Naive Bayes, Support Vector Machine, Random Forest, Convolutional Neural Network, BERT) were compared. The BERT-based methodology, which uses a state-of-the-art transformer structure and can consider the context of words in their classification due to its bidirectional capability, performed best on different evaluation metrics. The misclassifications were mostly in semantically adjacent categories, i.e. “very relevant” Tweets were sometimes misclassified as “rather relevant”.
So far, the methodology has only been applied to German-language tweets, as the primary case study was the Ahr Valley flood of 2021, which is a central use case in TEMA. In the future, however, this methodology is planned to be extended to other languages and topics (e.g. forest fires).
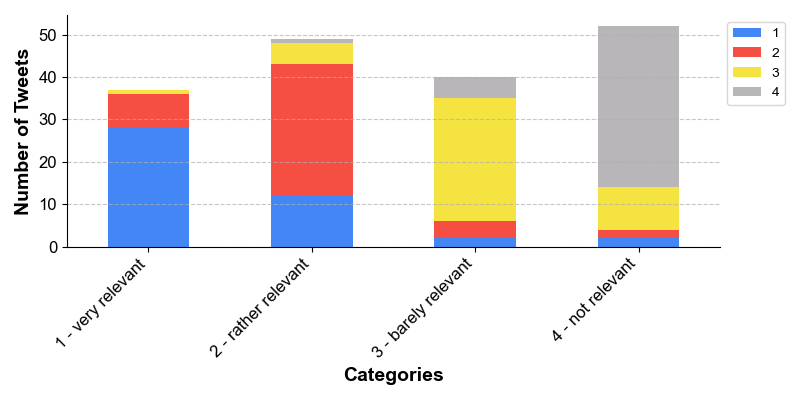
The corresponding paper „Drowning in the Information Flood: Machine Learning-Based Relevance Classification of Flood-Related Tweets for Disaster Management” by Eike Blomeier, Sebastian Schmidt and Bernd Resch has been published in Information and is available under https://www.mdpi.com/2078-2489/15/3/149.