Artificial Intelligence for Natural Disaster Management
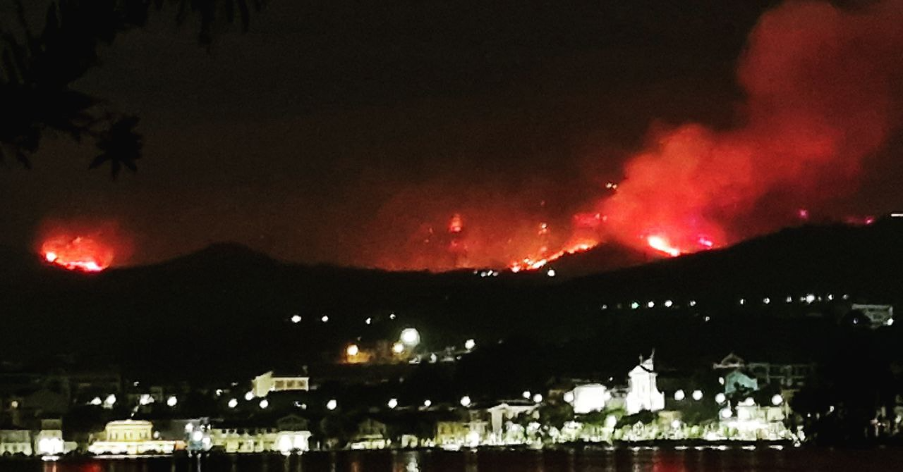
25 August 2023
Eng. Lorenzo Carnevale, Assistant Professor at UNIME
The climate is quickly changing. Almost every day we are informed by television and newspapers about a natural disaster. Just to mention what happened this summer, Greece [1] is enduring the hottest July of the last 50 years due to a heatwave longer than six days. Northern Italy [2] is living in a different situation. Hail as big as tennis balls, winds as fast as a supercar. The Milan sky became dark at midday and the hinterland was affected by a tornado. The Mediterranean [11] Sea reached its highest ever recorded temperature at over 28.71c, beating the previous record of 28.25C set in 2003. The summer will still have much to say.
In the meantime, researchers from academia and industry, along with the experts of the climate change field, are questioning how to prevent and mitigate the effects of such disasters. In this regard, this is not a mystery that the artificial intelligence (AI) is getting a momentum due to the popularity of large language model (LLM) solutions, such as the OpenAI’s ChatGPT, which led the G7 leaders [3] to call for “AI guardrails”. However, much more can be done for natural hazards. In this article, we wonder about the support that the AI may provide to disaster natural management.
The foundation of any AI-based methodologies is high-quality data [4]. However, the availability of data related to natural disasters is (let me say fortunately!) very limited due to the rarity of these events. In this regard, some solutions adopt the creation of synthetic data, which easily leads to unpredictable bias. Another possibility is represented by the transfer learning, which transfers the knowledge acquired from applying AI to a certain site and fine-tuned to another site [5]. When good data is available, AI can forecast isolated events, such as earthquakes [6] and wildfire [7]. Already in 2013 [8], AI and statistical models were used to evacuate 1 million people from the Bay of Bengal due to the very severe cyclonic storm “Phailin”. In 2019, the Pentagon and Carnegie Mellon University, along with Microsoft and the University of California, have collaborated to build xView2 [9], a tool that uses AI and satellite imagery to identify building and infrastructure damage in a disaster area and categorize its severity. Recently, this was used in Turkey and Syria to mitigate the frustration of citizens because of the delayed arrival of rescue after the devastating earthquake. Even in these successful cases, the modelling phase is critical because there are no standards to evaluate the algorithm and mostly to measure the accuracy if not when this could be too late. In the best case the results lead to false alarm but in the worst one the event is not predicted and prevention actions can not be taken in time.
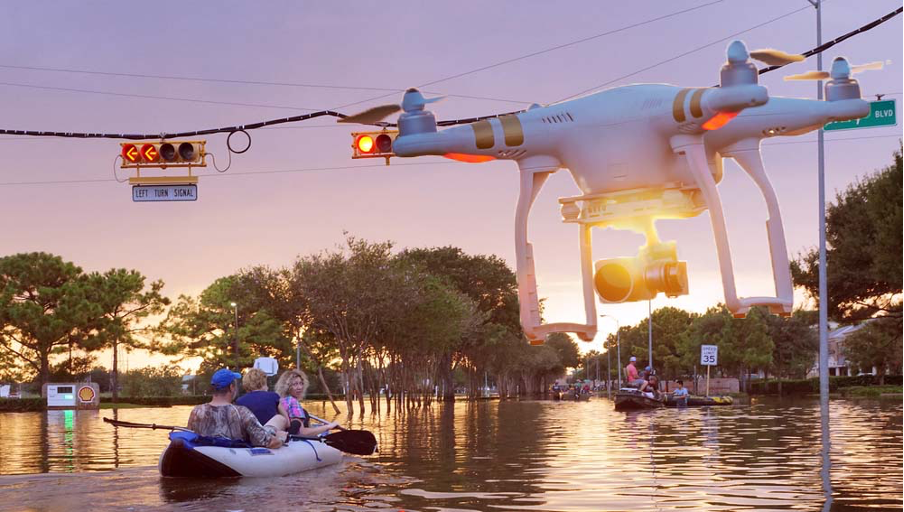
A great support to AI is provided by complementary technologies. Sensor networks are often deployed on crops, forests and remote areas thanks to long range wireless communication, powerful tiny devices and a cheap cost of materials. Big data are, therefore, collected in real-time and generate the datasets useful to build robust models. When stationary devices are not enough, autonomous unmanned vehicles, e.g., drones and rovers, can be involved for monitoring a target area or supporting the recovery operations. All these devices may bring AI capabilities to the edge of the network, where data are collected and events happen.
AI is therefore very promising in understanding, forecasting and communicating natural disasters, monitoring events in real time and anticipating specific risks, but further actions needs to be taken. Heterogeneous communities, such as Internet Technologies, geophysics, governing agencies and industries, need to share a common vision and skill all components in the field, e.g., control room operators and first responders. Algorithms need to become standard and common methods to evaluate the models need to be set up. Solutions should be built to favor reuse in different scenarios and contexts.
The Earth is ill and scientists are agreed that the major cause is the behaviour of human beings. The same are now called to take actions before it is too late.
Bibliography
- Greece faces hottest July weekend in 50 years, forecaster says, as scores of wildfires rage. The Guardian. https://www.theguardian.com/world/2023/jul/22/greece-faces-hottest-july-weekend-in-50-years-forecaster-says-as-scores-of-wildfires-rage
- Maltempo in Lombardia e nel Nord Italia: tornado e maxi grandinate. E il cielo di Milano diventa buio a mezzogiorno. Alessio Ribaudo, Corriere della Sera. https://milano.corriere.it/notizie/cronaca/23_luglio_22/maltempo-al-nord-tornado-maxi-grandinate-e-fulmini-e-il-cielo-di-milano-diventa-buio-a-mezzogiorno-640abea3-6283-4259-a2b6-c32d5de10xlk.shtml?refresh_ce
- The Path to Trustworthy AI: G7 Outcomes and Implications for Global AI Governance. Hiroki Habuka, Center for strategic & International Studies. https://www.csis.org/analysis/path-trustworthy-ai-g7-outcomes-and-implications-global-ai-governance
- Kuglitsch, M.M., Pelivan, I., Ceola, S. et al. Facilitating adoption of AI in natural disaster management through collaboration. Nat Commun 13, 1579 (2022). https://doi.org/10.1038/s41467-022-29285-6
- Jannes Münchmeyer and others, Earthquake magnitude and location estimation from real time seismic waveforms with a transformer network, Geophysical Journal International, Volume 226, Issue 2, August 2021, Pages 1086–1104, https://doi.org/10.1093/gji/ggab139
- R. Tehseen, M. S. Farooq and A. Abid, "EPS: An earthquake prediction system using Federated Learning," 2021 International Conference on Innovative Computing (ICIC), Lahore, Pakistan, 2021, pp. 1-8, doi: 10.1109/ICIC53490.2021.9692919
- A. E. Hoffy, S. Seok-Chul Kwon and H. -G. Yeh, "Federated/Deep Learning in UAV Networks for Wildfire Surveillance," 2023 Wireless Telecommunications Symposium (WTS), Boston, MA, USA, 2023, pp. 1-9, doi: 10.1109/WTS202356685.2023.10131685
- Mohanty, U. C., and Coauthors, 2015: A Great Escape from the Bay of Bengal “Super Sapphire–Phailin” Tropical Cyclone: A Case of Improved Weather Forecast and Societal Response for Disaster Mitigation. Earth Interact., 19, 1–11, https://doi.org/10.1175/EI-D-14-0032.1
- Computer Vision for Building Damage Assessment. xView2. https://xview2.org
- Houston Flood Chance for Drones to Shine. Bold Insights. https://www.boldbusiness.com/digital/drone-use-in-houston-flood
- Mediterranean Sea breaks new heat record: What does this mean for weather in Europe? Daniel Harper, Euronews. https://www.euronews.com/green/2023/07/26/mediterranean-sea-breaks-new-heat-record-what-does-this-mean-for-weather-in-europe