Adjusting the Rate of Spread of Fire Simulations in Real-time
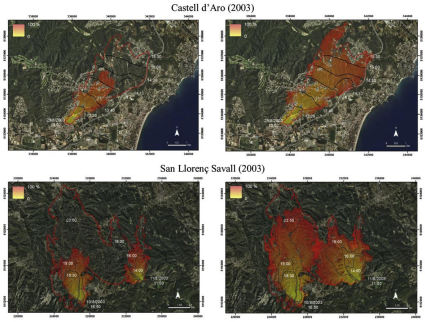
14 March 2024
Adrián Cardil Forradellas, PhD., Senior Fire Modelling Scientist at Tecnosylva.
Catastrophic wildfires occurring under extreme weather conditions challenge fire suppression capabilities in many countries. Every year millions of hectares of forest are burned, damaging and destroying human settlements and ecosystems, and putting at risk human lives [1].
Fire simulation and modelling allow for characterising and predicting fire spread and behaviour in diverse and complex fire conditions [2][3]. Fire management and suppression agencies rely on fire propagation simulation models during operations to analyse fire incidents in real time and support fire suppression strategies and tactics [4]. Most simulators can predict fire spread and behaviour forward in time (propagation) to estimate the rate of spread (ROS), flame length or minimum travel time paths with a relatively high degree of accuracy using variables such as ignition location, fuel type, fuel moisture, weather and topography [5]. The capability of accurately predicting fire spread is associated with uncertainties that may undermine the utility of such predictions for real-time operational fire suppression decision-making. This uncertainty may come from limitations and assumptions in fire models [6], complex spread processes such as spotting and active crown fire, variability in topography that influences wind fields, availability and quality of input data and local fuel calibration [7]. To provide reliable information to fire managers, these uncertainties must be addressed. Rothermel and Rinehart [8] and Finney [9] proposed adjustment factors of ROS to adjust simulated fire growth to real fire spread in Behave and Farsite fire simulators, respectively. These factors are fuel model specific and are included as input data in equations of the fire spread model, multiplying the initial ROS by the adjustment factor. Fire analysts assessed these adjustment factors manually, trying to find the best adjustments iteratively. In addition to the errors derived from analysts conducting a manual process, it was a very time-consuming task and difficult to be applied in an emergency.
In this study, fire growth simulations and their adjustments were done using Wildfire Analyst (WFA) [4]. WFA is a software that provides real-time analysis of wildfire behaviour and simulates the spread of wildfires to directly support multi-agency wildfire incident management.
After the revision of the potential sources of divergence between the simulated fire growth and real fire spread, WFA can be used to adjust simulated fire growth to the observed fire progression in order to provide more accurate and reliable estimations of future fire behaviour using ROS adjustment factors. Therefore, WFA includes a data assimilation technique capable of quickly adjusting simulations to observed fire behaviour. The strength of the method is that it is not recursive and may be solved almost instantaneously, providing a very clear and easy way to interpret adjustments for fire managers at an operational level, and that it requires very few inputs [4]. Adjustment data can consist of a set of control points or lines where the arrival time of the real fire is known. The method is based on determining the best ROS adjustment factors [10] using a least squares approach to minimize the error between the simulated fire growth and the real fire. Mathematically, the method searches for the optimal adjustment factors that multiply the initial ROS of the simulated fire over each fuel type.
In order to show the adjustment method, two case studies were used in Catalonia: Castell d’Aro and San Llorenç Savall wildfires occurred in 2003. Fire propagation simulations were adjusted using the fire monitoring by firefighters.
As shown in Figure 1, fire simulations by using Rothermel [8] equations without adjustments for Castell d’Aro and San Llorenç Savall fires underestimated ROS. However, the adjustment mode of WFA greatly improved the outcomes, with final simulated fire spread aligning well with field observations, and significantly diminishing both burned area and time errors. The case studies showed how this mode helps reconstruct more accurately past fires and analysing fire spread and behaviour.
Figure 1. Time elapsed of free simulated fire growth (left) and adjusted simulated fire growth (right) considering the adjustment factors.
The method is especially useful in suppression situations, where providing more accurate estimates of fire spread can be critically important for the development of strategy and tactics. The algorithms may be solved almost instantaneously and as shown in the case studies, the adjustment mode of WFA could be used for a better prediction of fire spread.
The adjustment approach is not limited to measurements by firefighters in real-time (Castell d’Aro and San Llorenç Savall fires). Wildland fire growth simulation adjustment can be done through other sources that provide the fire location at a given time. Data from Unmanned Aerial Vehicles (UAVs), GPS locations from suppression resources or satellite active fire data may be useful data to adjust fires, even in a remote way.
In TEMA project, the position of fire fronts will be continuously updated by UAVs and satellite images, providing the actual position of the fire front at each moment of the fire. This real position could be used to feed the fire simulator with accurate information to adjust the rate of spread of fire simulations in real time.
References
[1] Cardil, A., Delogu, G.M., Molina-Terrén, D.M., 2017. Fatalities in Wildland Fires from 1945 to 2015 in Sardinia (Italy). Cerne 23, 175–184. https://doi.org/10.1590/01047760201723022266.
[2] Albini, F., 1976. Estimating Wildfire Behavior and Effects. Intermountain Forest and Range Experiment Station, USDA Forest Service General Technical Report. INT-30. Utah.
[3] Finney, M., 2002. Fire growth using minimum travel time methods. Can. J. For. Res. 32, 1420–1424. https://doi.org/10.1139/X02-068.
[4] Ramirez, J., Monedero, S., Buckley, D., 2011. New approaches in fire simulations analysis with Wildfire Analyst. The 5th International Wildland Fire Conference.
[5]Alcasena, F.J., Salis, M., Vega-Garcia, C., 2016. A fire modeling approach to assess wildfire exposure of valued resources in central Navarra, Spain. Eur. J. For. Res. 135, 87–107. https://doi.org/10.1007/s10342-015-0919-6.
[6] Rodríguez y Silva, F., Molina-Martínez, J., 2012. Modeling Mediterranean forest fuels by integrating field data and mapping tools. Eur. J. For. Res. 131, 571–582. https://doi.org/10.1007/s10342-011-0532-2.
[7] Benali, A., Sá, A.C.L., Ervilha, A.R., Trigo, R.M., Fernandes, P.M., Pereira, J.M.C., 2017. Fire spread predictions: sweeping uncertainty under the rug. Sci. Total Environ. 592, 187–196. https://doi.org/10.1016/j.scitotenv.2017.03.106.
[8] Rothermel, R., 1972. A mathematical model for predicting fire spread in wildland fuels. USDA For. Serv. Intermt. For. Range Exp. Stn. Res. Pap. INT-115. Ogden, UT.
[9]Finney, M.A., 1998. FARSITE, Fire Area Simulator–model Development and Evaluation. Research Paper RMRS-RP-4 Revised. U.S. Department of Agriculture, Forest Service, Rocky Mountain Research Station, Ogden, UT.
[10] Rothermel, R., Rinehart, G., 1983. Field procedures for verification and adjustment of fire behavior predictions. Gen. Tech. Rep. INT-142. Ogden, UT U.S. Dep. Agric. For. Serv. lntermountain For. Range Exp. Stn., pp. 25.